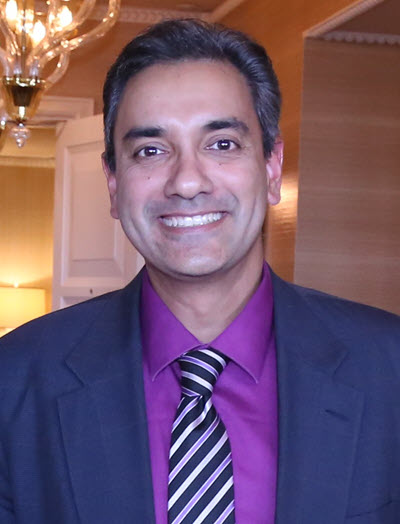
Though still mainly confined to the research space, new artificial intelligence (AI) and digital pathology solutions are slowly entering the clinical space. However, while these solutions have great power and potential to improve patient care, there are also possible pitfalls.
Rajesh C. Dash, MD, is a Duke Health pathologist, chair of the College of American Pathologists’ Artificial Intelligence Committee, and panelist on the Panel of National Pathology Leaders. Here, he discusses recent developments in AI and digital pathology, as well as associated challenges and potential solutions around what pathologists and other lab professionals can do to prepare for bringing these technologies into their labs.
Q: How are pathologists currently using AI and digital pathology solutions?
A: In terms of AI, the use of machine learning [ML] has been expanding in the field of pathology, not just in the imaging space, but in the clinical laboratory as well. The very first FDA-approved surgical pathology algorithm was approved on September 21, 2021. That was Paige’s algorithm for detection of prostate cancer as a QC/QA tool (not for primary diagnosis of prostate cancer). The FDA approval is only for identifying tumors that pathologists might have missed, making it more of a quality check than a diagnostic algorithm in the way it’s currently marketed.
Digital pathology has been slow to move forward for a number of reasons. Recently, it has accelerated, in part due to COVID and the need for remote sign out, i.e., case finalization following glass or digital slide review. With COVID, the FDA and CLIA have relaxed some of the requirements for where a diagnosis may be made, and that has helped accelerate digital pathology.
Q: Why haven’t AI and digital pathology solutions been more widely adopted?
A: Part of the challenge is that there’s a significant cost for implementing digital pathology on top of all the other work that needs to happen in traditional pathology, which is signing out using a microscope, or looking at a glass slide with tissue embedded between glass. Glass slide diagnostics have certain costs associated with them, and you don’t save anything by going digital. You have to incur the cost of a digital scanner, the cost of the person that’s trained and uses that digital scanner, and the quality assurance of making sure you’re scanning everything on the glass slide and that it’s in focus across all the different tissue fragments that might be on that slide. Then, that information needs to be attached to that case somehow in the electronic medical record, so the pathologist can review it.
Generally speaking, most pathologists have indicated that systems are immature and the sign out (slide review) process is inefficient. There have been a few places outside the US that are reporting in literature that, after some training and experience, the efficiency increases to a point where it’s actually faster than glass slides. That’s debatable as there are many variables that might affect slide review efficiency.
What most folks believe in this space is that, eventually, the value-added services built on top of digital pathology, such as AI, will raise the standard of care and provide the type of workflow efficiency that makes it worthwhile to invest in the technology. That’s the reason that, in 2021, the College of American Pathologists decided to prepare for this and create the Artificial Intelligence Committee, which I now chair. We have written a strategy around how we think this technology is going to impact the field and how we need to prepare for the change in technology and prepare our members to be ready for when it does start to significantly impact our specialty.
AI has many skeptics that have written about the biases around it. There’s legislation in Europe about precluding facial recognition so that patient privacy isn’t invaded. In the pathology space, there’s a risk of training these AI/ML models in a way that skews the results toward a certain population, being accurate in a certain population but not in others. AI must be validated to work well in a particular laboratory and then must be monitored over time to ensure that performance is maintained.
Q: How can pathologists overcome these challenges?
A: Some of the solutions really revolve around working together as a community with the subject matter experts, the vendors, the technology experts, providing thought leadership on the strengths, weaknesses, opportunities, threats, and risks, and coming up with a plan to address them by being proactive. Hopefully, we can optimize for the benefits that these new technologies are supposed to afford our patients and providers and mitigate the risks.
Q: How are AI and digital pathology solutions evolving?
A: The development is really in the research space. There are very few algorithms that are FDA approved, though there are two cytopathology algorithms that have been FDA approved for use in screening pap smears for over a decade. They’ve been very successful; the risks haven’t really come to light in terms of patient safety. But both of those systems have a very locked down process, and the algorithms don’t change.
One of the purported benefits of AI is that it can adapt and learn over time, so it continues to get better. With that type of technology, which is very powerful, there’s also the opportunity to mislearn and make mistakes. So, the question is, how do you monitor the performance of learning to ensure that it’s a proper learning? How do you identify mistakes in these systems when professionals do make them—what type of quality control procedures do we need to have in place?
In terms of technology, there’ll be more options out there for scanners that are not as expensive as the ones we have today. That should help to accelerate the digitization of anatomic pathology by providing a lot of flexibility in terms of where these cases are signed out, how quickly they can be signed out, and the ability to integrate information.
Q: What advice do you have for pathologists in terms of preparing for these technologies?
A: Stay in touch with the specialty societies that are trying to prepare you. For this space, there are a lot of educational programs out there to understand not only what benefits this technology brings your practice, but also the risks so that laboratory directors can implement these technologies in ways that are very similar to the way laboratory tests might be implemented. These programs offer a step-by-step process that clarifies how to go about validating that these technologies are safe to use in your laboratory, that they’re efficacious, and that their performance remains consistent over time.
Q: How do you see AI and digital pathology changing going forward?
A: The FDA has drawn a line regarding AI, ML, and software as a medical device. They clearly see these technologies as potential patient safety issues. That’s probably not going to change and will slow things down, because they want to make sure there aren’t products in the market that are adversely affecting patient care. So, there’s no need to be concerned about a sudden onslaught of new products where a consumer is unable to decide, or is fraught with multiple perilous decisions that might impact care of their patients. There’s going to be an opportunity for the FDA to weigh in, but there’s also going to be opportunity for laboratory directors to say, “Hey, there’s a new technology out there that might save my practice time.” In the setting of financial constraints and reducing reimbursements, it might be very attractive to implement some of these technologies.
The lesson to be learned there is that you’ve got to be careful and really understand what you’re getting into. Make sure you’re aware of the risks, the challenges, and procedures you need to look at to make sure these technologies don’t have unforeseen unintended consequences. That’s the role that laboratory directors play before implementing any new instrument, technology, or test in their laboratory.
Q: Did you have anything more to add?
A: There are technologies being deployed that leverage laboratory data but that are not in the laboratory space, so it’s a little unclear who would monitor that. The Epic sepsis algorithm is a good example of that. It leverages some laboratory data that are predictors of a patient being septic and tries to predict which patients require intervention and hopefully get interventions earlier before they get too sick. The algorithm has been criticized heavily for not working very well.
But that hospital space is not regulated in the same way that the laboratory space is with CLIA. I don’t think that this is widely known or recognized. I do think that the FDA is probably now recognizing that, but they haven’t proposed anything that differentiates software as a medical device in the laboratory space versus not in the laboratory space. That’s something that might become clearer in the future.
It’s also unclear how the technology would be reimbursed. So, what is the incentive for laboratories to implement some of these newer technologies when all they do is cost more money at this point in time? There’s currently no revenue associated with using AI in the healthcare space. So, it’s unclear if the charge capture mechanisms for services rendered in healthcare will eventually accommodate the use of new technologies, particularly if those new technologies are proving to either be safer or more efficacious than traditional methods.