AI: The Newest Member of the Lab Team?
AI is an increasingly useful tool in pathology—but its full value can only be realized in collaboration with human experts.
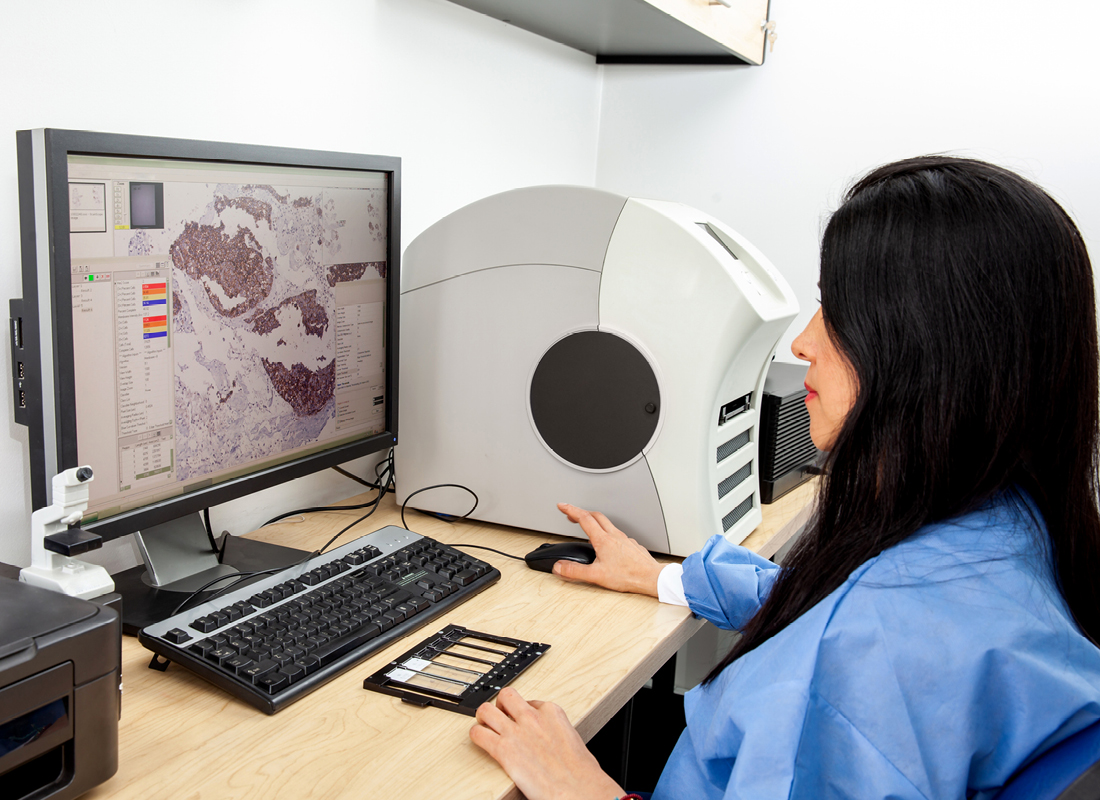
Pathology is in the midst of a digital transformation—not just from traditional glass slides to computer-based images, but also from traditional diagnostic processes to those supported by artificial intelligence (AI). A recent survey of international experts in digital and computational pathology revealed strong agreement that AI will rapidly change the face of pathology, increasing diagnostic accuracy and automating time-consuming or burdensome tasks to alleviate heavy workloads.1 What is less certain is how the field will reach that point. Where in the laboratory will AI confer the most benefit? Where does it carry the fewest risks? And how can laboratory professionals best integrate AI into their existing workflows?
Recent advances
With new developments in computing and AI technology, the role of computational pathology is expanding. A recent review divided AI-based tools into those that contribute to a diagnosis (or a prediction or prognosis) and those that assist with laboratory work.2 The former category includes triaging slides for pathologist examination, analyzing the characteristics of the tumor microenvironment, or even combining histologic and genomic information to help select treatments or predict patient outcomes.3 The latter includes cell counting, nucleic acid or immunohistochemistry quantification, automated biomarker requests, and more.4 Multiple instance learning, a type of supervised machine learning, is a growing presence on the pathology horizon because of its ability to assist with predicting clinical outcomes even without fully annotated data2—but there’s still a need for bigger, better datasets and more advanced technologies before AI can fully realize its clinical potential.
Computational collaboration
An AI Glossary
Algorithm: the set of instructions an AI model follows to perform its task
Artificial intelligence: a program’s ability to perform tasks in a way that mimics human intelligence
Convolutional neural network (CNN): a type of neural network that includes at least one layer whose purpose is to reduce the overall complexity of the input data
Deep learning: a type of machine learning in which computers analyze data in multiple layers to extract progressively more complex information
Foundation model: a type of generative AI that is self-trained on massive quantities of data, generates output in response to natural language instructions, and has a wide range of applications
Generative AI: a type of AI that can learn from training data and generate new data of the same type (for instance, text or images)
Large language model (LLM): a type of neural network that can perform natural language processing tasks such as responding to questions or describing complex data in understandable terms
Machine learning: a type of AI in which computers analyze data and use the resulting information to develop and refine their algorithms, rather than requiring a human to program them
Model: a program that carries out a specific task, such as analyzing a pathology slide and making predictions based on patterns it has observed in previous slides
Natural language: a human language that has developed naturally (such as English), rather than one that has been artificially constructed (such as Esperanto or programming languages)
Neural network: a type of machine learning model that analyzes training data and generalizes the resulting knowledge to new data; its name comes from its organization into a group of interconnected “nodes,” inspired by neuronal organization in the human brain
In research, however, the promise of AI is already evident. A study from the Clinical Proteomic Tumor Analysis Consortium (CPTAC) developed an integrative approach that combines histopathology, transcriptomics, proteomics, and deep learning to predict clinical outcomes across a range of cancers.5 Using a database of 2,755 well-annotated hematoxylin- and eosin-stained slides from six cancer types, the researchers asked their convolutional neural network (CNN) models to perform a range of diagnostic tasks. The models demonstrated high accuracy in predicting tumor tissue of origin—and, although not trained to do so, in distinguishing tumor from normal adjacent tissue. Further investigation revealed that the models paid more attention to tumor regions than to healthy tissue, giving particular weight to the size, shape, and density of nuclei within each tile.
The more complex decisions the CNN models made, such as biomarker or outcome predictions, fell into two groups: those in which humans could intuit the features used and those in which they could not. Both groups are useful—the first potentially opens up new avenues for tumor classification and the second suggests areas in which AI-based support can enhance diagnostics beyond what humans alone can accomplish. In the CPTAC study, a model trained on squamous cell carcinoma of the lung was able to identify tumor tissue in clear cell renal cell carcinoma even though human pathologists could not ascertain features specific to those two cancer types. The models even predicted molecular characteristics from histology, identifying areas of the slides that suggested enrichment in genes and proteins related to DNA replication or repair and using specific cell and tissue features to predict common mutations in genes such as EGFR, KRAS, PTEN, STK11, and TP53.
The most fascinating angle of this study is its focus on human-computer integration. By seeing the features the algorithm gave most attention, the authors could identify potential histologic clues to cancer type, grade, stage, and even molecular profile that might otherwise have gone unobserved. Incorporating expert pathologist review also allowed them to better understand the CNN models’ behavior and how AI-based diagnostic support can assist laboratory medicine professionals without overstepping its bounds.
The future of diagnostic AI
“The job of a pathologist is complex and they need to take away the fear that AI can replace them,” says computational pathology expert Rajendra Singh, MD, director of dermatopathology at Northwell Health and a Digital Pathology Association board member. He offers an example from his own practice. “I look at 100 cases every day. Of those, one or two are melanomas with which AI can help. The majority are dysplastic nevi, basal cells, and squamous cells in which AI has not been proven. For the rest of the cases, there is currently no role for AI. So, for a small number of cases, AI is truly helpful—but these are publicized so heavily that they create fear. It’s better to think of AI as another tool, like immunohistochemistry, that can help us do our jobs better.”
With that said, Singh sees the value of AI-based diagnostic support—and encourages labs to get on board early. “There will be a time when you are going to get a phone call from a patient or clinician demanding a digital slide so they can run the algorithms,” he says. “At that time, you will have to scramble to put in the infrastructure to go digital. It would be prudent for pathology departments to go digital now when they can plan the entire process and build it in a way that it helps the pathologists and the department and lets them keep control of the data.”
So what should laboratorians—and labs—be doing now to prepare? Singh says, “Validation is key, so putting protocols in place, building case sets that can be used for validation, and having standards to compare against are critical. Out-of-domain samples (such as prostate samples with lymphoma when you are testing for Gleason score in prostate adenocarcinoma) will also be needed.” He further cautions that quality will be key. “Garbage in, garbage out. A whole slide image is only as good as the quality of the glass slide, so ensuring that the lab can produce high-quality glass slides is critical for getting any value out of these models.”
For the prepared lab, Singh believes the future of computer-aided diagnostics is bright—and he’s particularly looking forward to the next generation of lab AI. “The current crop of AI models will soon be relegated to AI 1.0 because they were built with one type of data. The next generation of AI models will be built using multimodal inputs, including foundation models, and will be more robust and practical.”
References:
- Berbís MA et al. Computational pathology in 2030: a Delphi study forecasting the role of AI in pathology within the next decade. EBioMedicine. 2023;88:104427. doi:10.1016/j.ebiom.2022.104427.
- Song AH et al. Artificial intelligence for digital and computational pathology. Nat Rev Bioeng. 2023. doi:10.1038/s44222-023-00096-8.
- Kim I et al. Application of artificial intelligence in pathology: trends and challenges. Diagnostics (Basel). 2022;12(11):2794. doi:10.3390/diagnostics12112794.
- Rakha EA et al. Current and future applications of artificial intelligence in pathology: a clinical perspective. J Clin Pathol. 2021;74(7):409–414. doi:10.1136/jclinpath-2020-206908.
- Wang JM et al. Deep learning integrates histopathology and proteogenomics at a pan-cancer level. Cell Rep Med. 2023;4(9):101173. doi:10.1016/j.xcrm.2023.101173.
Subscribe to Clinical Diagnostics Insider to view
Start a Free Trial for immediate access to this article