AI Using Laboratory Data to Inform Clinical Decisions
From - Diagnostic Testing & Emerging Technologies Artificial intelligence- (AI-) based technology is beginning to penetrate health care. Like most other clinical decision-making, several early AI applications rely upon… . . . read more
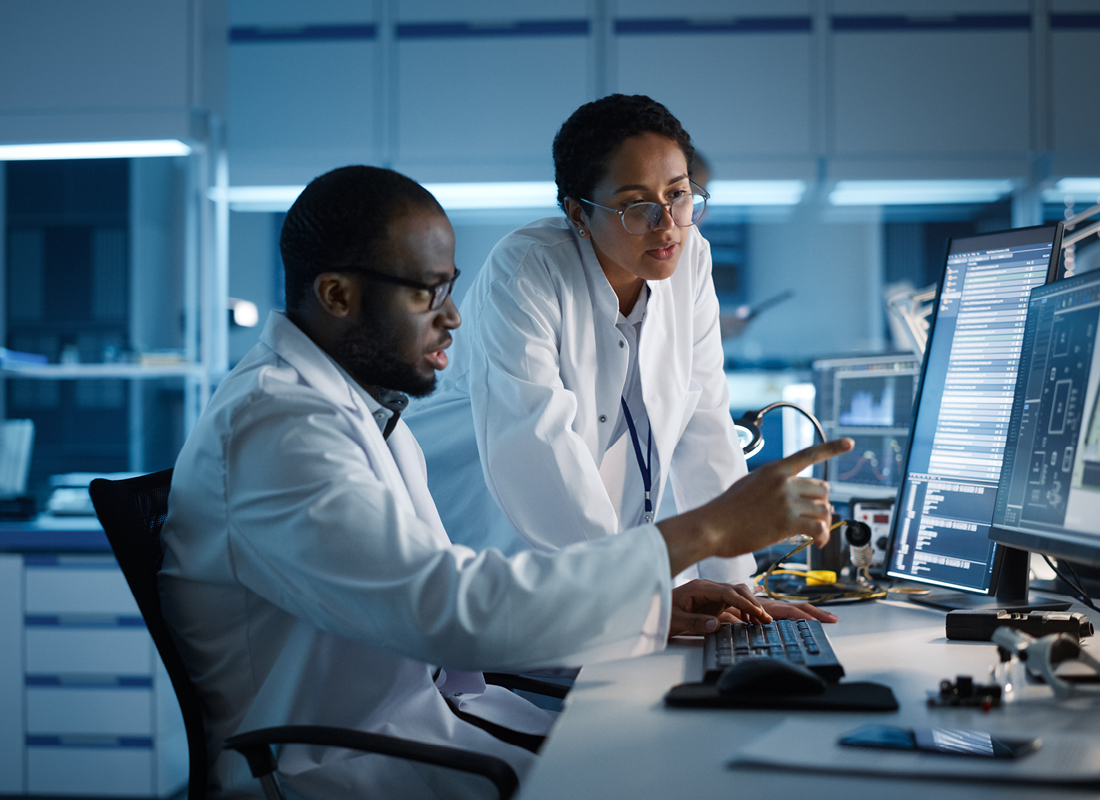
Artificial intelligence- (AI-) based technology is beginning to penetrate health care. Like most other clinical decision-making, several early AI applications rely upon laboratory data.
While personalized medicine is often thought of in tandem with genomic medicine, genomics is not the only data source to inform AI-based algorithms. Other biological high throughput data (transcriptomics, epigenomics, proteomics, metabolomics) can impact clinical decision-making, but so can imaging, electronic health records (EHRs), health claims data, and data from wearable sensors or mobile health apps.
While there are cases where a single marker can stratify patient populations (e.g., Herceptin and HER2), most diseases are complex—either impacting multiple biological pathways or with a unique interplay between biological and environmental or lifestyle factors. Understanding of these multifactoral signatures requires processing of complex, high-throughput data, which is increasingly done with AI.
Several new studies show that laboratory test results, including genetic sequencing data, can be successfully incorporated into AI algorithms to predict disease risk and treatment response.
AI Predicts Aneurysm Risk With Genomic Data
A new algorithm that incorporates genome-sequence data and electronic health records can predict an individual’s risk of abdominal aortic aneurysm (AAA) based on the interaction of genetic susceptibility and lifestyle factors, according to a proof-of-principle study published Sept. 6 in Cell. This platform can potentially be used to develop clinical tests for other complex diseases, as well as personalized health management and disease intervention.
AAA affects an estimated 3 million people each year, the authors say, and is the 10th leading cause of death in the United States. It often develops asymptomatically, with the aorta silently enlarging, until it bursts, with often deadly consequences. There is currently no early screening test.
The authors say AAA was an ideal condition to target using the AI technology because it has a strong genetic component (an estimated heritability of 70 percent), yet its genetic underpinnings are not understood and there are “robust” associations between clinical outcomes and lifestyles.
The Stanford University researchers performed high-coverage, whole-genome sequencing (WGS; average genome coverage of 50×) for 268 AAA patients and 133 controls seen at the Veterans Affairs Palo Alto Healthcare System, Stanford University, and Kaiser Permanente. They devised a novel machine-learning framework with a cloud-based analytical pipeline that was able to process large amounts of personal genomes and electronic health record data.
At the individual variant level, the algorithm, called Hierarchical Estimate From Agnostic Learning (HEAL), examined the potential clinical relevance of each individual mutation by annotating its functional consequences, population frequency, and the predicted pathogenicity. In total, 66,047 rare, nonsynonymous mutations (missense, nonsense, and those affecting splice sites) that were not present in the European populations analyzed in the 1000 Genomes Project were identified.
The machine-learning framework agnostically identified a subset of 60 genes showing distinct mutational patterns capable of distinguishing cases from controls and used this pattern to predict clinical outcomes from personal genomes. The mutations implicated 40 distinct functional pathways that played roles in blood-vessel function and aneurysm development, as well as some more surprising functions, such as regulation of immune function. In validation analysis, these
AAA-related mutational patterns were not seen among healthy individuals.
Using genetic sequence data only, HEAL could accurately predict with about 70 percent accuracy who is at high risk for AAA. But, with the addition of EHR information from their last clinical visit (e.g., sex, age, status as a regular smoker, heart rate, waist-to-hip ratio, insulin level, fasting glucose level, lipid profiles), HEAL’s predictive abilities improved to nearly 80 percent, a level similar to or better than many existing clinical screening tests like those used for gestational diabetes, significant coronary stenosis, and cytology-based cervical cancer screening, the authors say.
“Our study suggests that HEAL could be potentially developed into a clinically actionable test for early screening of AAA,” writes senior author Michael Snyder, Ph.D., from Stanford University. “By simultaneously modeling individual genomes and personal EHRs, HEAL revealed the interplay between the personal genome baseline and individual lifestyles underlying AAA predisposition, demonstrating its potential as a highly personalized health management tool.”
The authors acknowledge that the genome-based model has a “low” false-negative rate, but a “relatively higher” false-positive rate. They add, though, that early assessment for disease risk is “critical,” given the disease’s irreversible progression and that inexpensive and noninvasive ultrasound can be used as follow-ups for positive results.
In their next phase of work, the research group is looking to apply HEAL to detect the genetic underpinnings of preterm birth and autism.
Takeaway: AI is enabling better understanding of disease pathology through examination of complex data sources. The HEAL platform has been successfully applied to AAA risk and may become a screening test or personalized health management tool for other complex conditions.
Subscribe to Clinical Diagnostics Insider to view
Start a Free Trial for immediate access to this article