AI: With the Lab Every Step of the Way
Artificial intelligence models have a valuable role to play in each phase of the total testing process.
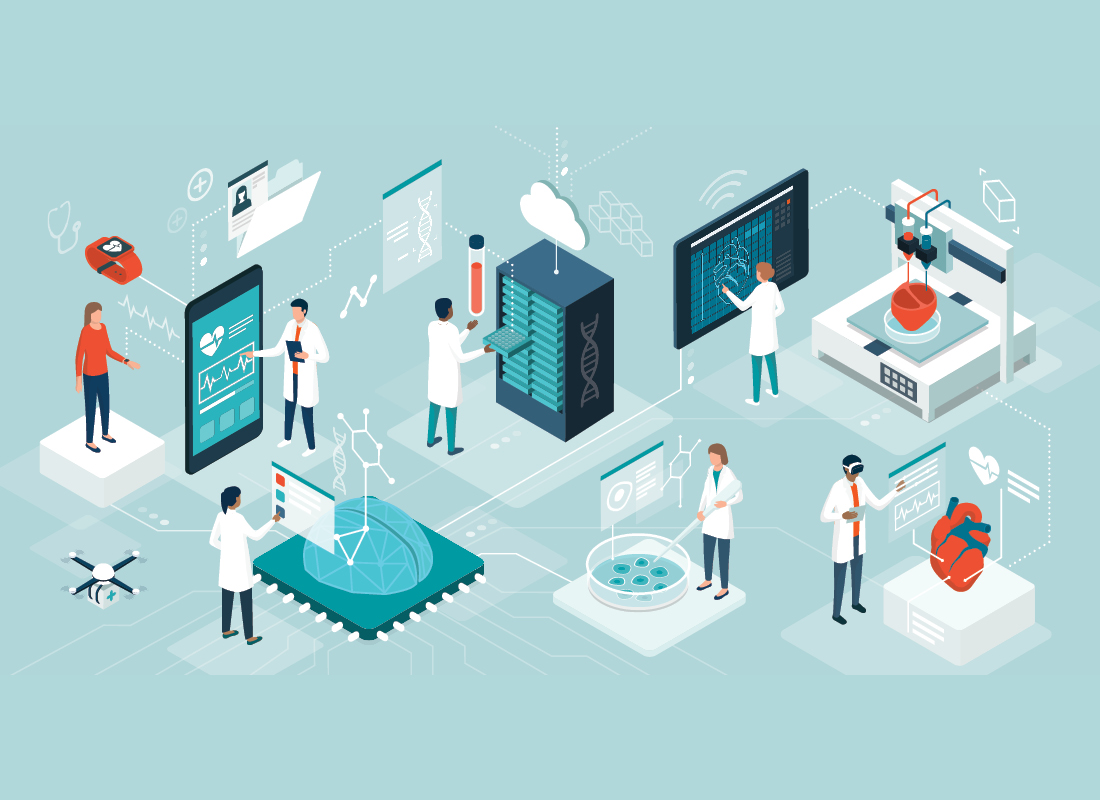
Artificial intelligence (AI) is an increasing presence in many laboratories. From automating cell counts to screening large amounts of genomic data, AI models can take on time-consuming tasks and allow pathologists and laboratory medicine professionals to focus on complex demands. But though AI is most recognized for the support it provides during the analytical phase, the technology has a role to play at every stage of the total testing process.
Lab AI—now and in the future
“I’ve always said that the full value of AI models will emerge when they really help patients and clinicians,” says Rajendra Singh, a dermatopathologist and digital pathology expert who sits on the College of American Pathologists’ Digital and Computational Pathology Committee and on the Board of the Digital Pathology Association. “For the last century, pathologists have done a good job of making accurate diagnoses,” Singh says. “AI can accelerate diagnosis but, for most cases, speed is not essential. It is the quality of the diagnosis that is critical.”
Newer diagnostic models based on convolutional neural networks now predict the genomic changes underlying disease, which can act as prognostic tools or as companion diagnostics to help identify the treatments with the highest likelihood of success. “Some companies have developed drugs that can target HER2-low cancers,” Singh elaborates. “Multiple AI models can predict which patients have HER2-low cancers. Those models can now help match patients with the right treatment. I think that is a major change from where we were just a few years ago.”
Computer vision models are another promising avenue for the lab. These models are trained on both images and context (such as captions or pathology reports) with the goal of developing tools that can use these types of information to determine diagnoses and prognoses. A number of recent studies have focused on developing these tools, including one that trained its model on images from X (formerly Twitter),1 another that used information from PubMed and textbooks,2 and an ongoing collaboration between Microsoft and Paige using whole slide images to produce the largest image-based AI pathology model to date.3 “We are going to use computer vision models based on generative AI to predict patients’ diagnoses or prognoses,” says Singh, “and I think that is the most exciting part right now.”
AI throughout the total testing process
“The best way to look at key growth areas for AI is by preanalytical, analytical, and postanalytical phases,” Singh explains.
Preanalytical AI
In the preanalytical phase, AI tools can examine digital images and determine whether they are of sufficient quality for diagnostic use. “That is the first critical step where AI models are going to be very important—making sure that the quality is good enough to help make a diagnosis.” Singh also believes that large language models have a lot to offer in terms of summarizing patient history and other case details for easy access. “Just yesterday, a colleague asked me, ‘Do you think this is a melanoma?’ When I looked at this slide, I saw a little bit of fibrosis in the dermis, which indicated that there might have been a previous biopsy on the site. Because the clinician had not mentioned it, the pathologist hadn’t realized until we went back into the records and found it. Because there had been a previous biopsy and recurrent lesions can look similar or atypical, the diagnosis became ‘recurrent melanoma’—a significant change. If there had been a textual AI model to look into the patient’s clinical history and alert the physician or the pathologist to the previous biopsy, it would have quickly prevented an incorrect diagnosis.” Having AI models in the preanalytical phase of testing scan the patient’s history and provide the gist of it can help prevent errors and support pathologists and clinicians in diagnosing and treating patients more effectively.
Analytical AI
In the analytical phase, AI models can help pathologists examine slides for diagnostic purposes and compare them with previous samples to evaluate disease progress. Algorithms that incorporate elements of flexibility and learning can also offer more accurate predictions of values that are difficult, costly, or time-consuming to measure, such as low-density lipoprotein cholesterol or ferritin levels.4 AI can also support improvements in automation, classification, and data processing and analysis.5
Postanalytical AI
The postanalytical phase involves interpreting test results, delivering actionable clinical information, and forecasting future outcomes and issues. “In the postanalytical phase, AI models can help with prognosis or with predicting treatment outcomes,” says Singh. Machine learning has been used to improve the diagnostic value of biomarkers in combination,6 assess patient prognoses,7 and even perform prenatal screening for genetic disorders.8 Textual models can also help generate reports, a feature Singh appreciates. “In my practice, junior pathologists constantly come in and ask, ‘Can you look at this report? Does it contain the right information?’ The clinician is not looking at the slide, so the report needs to give them (and the patient) everything they need.” Pathologists and laboratory medicine professionals must be trained to clearly communicate information and provide appropriate context—and large language models can help. “One small error can completely change the context of a report. That happens in my real-life practice. So these models can have a big impact throughout the total testing process.”
Accelerating AI adoption
Our available computing power has increased exponentially in recent years—and the quality and quantity of data have improved correspondingly. “The data we have now is of a much higher quality than what we had five years ago,” says Singh. “It’s also multimodal now; data from radiology, pathology, electronic health records…all that is now available to clinicians and researchers.” Cloud resources are also facilitating data sharing for remote consultations or education. “If I want to send slides to someone else, it takes one click—and they are equally easy to download. Then you can annotate that data (or, if you’re a computer scientist, you can hire a pathologist to do it). Cloud computing and the ease of sharing information have made our work much easier.” Each technological advance contributes to the growth of the field—but, taken together, it’s clear how quickly AI’s role in the lab is expanding.
But Singh emphasizes that, despite this rapid growth, laboratorians remain at the heart of the discipline. “There are over 8,000 diagnoses in pathology. How do you build a model that can accurately differentiate between 8,000 potential diagnoses? And, if you build one, how will you integrate it into the lab? The narrow AIs will always provide the best support in the laboratory. The field is so vast that it’s currently impossible to artificially replicate what I do in even one day’s work. Instead, we need to start thinking of AI as a companion or a ‘copilot’ in the lab—a tool to help us do our jobs more effectively.”
References:
-
- Huang Z et al. A visual-language foundation model for pathology image analysis using medical Twitter. Nat Med. 2023;29(9):2307–2316. doi:10.1038/s41591-023-02504-3.
-
- Chen RJ et al. A general-purpose self-supervised model for computational pathology. ArXiv. 2023. doi:10.48550/arXiv.2308.15474.
-
- Paige. Paige Announces Collaboration with Microsoft to Build the World’s Largest Image-Based AI Model to Fight Cancer. September 7, 2023. https://paige.ai/paige-announces-collaboration-with-microsoft-to-build-the-worlds-largest-image-based-ai-model-to-fight-cancer/.
-
- Zhang L, Hu Z-D. Clinical applications of machine learning in pre-analytical, analytical and post-analytical phases of laboratory medicine: a narrative review. AME Med J. 2022;7:7424. doi:10.21037/amj-22-92.
-
- Yang HS et al. Building the model. Arch Pathol Lab Med. 2023;147(7):826–836. doi:10.5858/arpa.2021-0635-RA.
-
- Ren Z et al. Identifying tuberculous pleural effusion using artificial intelligence machine learning algorithms. Respir Res. 2019;20(1):220. doi:10.1186/s12931-019-1197-5.
-
- Chan L et al. Derivation and validation of a machine learning risk score using biomarker and electronic patient data to predict progression of diabetic kidney disease. Diabetologia. 2021;64(7):1504–1515. doi:10.1007/s00125-021-05444-0.
-
- He F et al. A machine learning model for the prediction of down syndrome in second trimester antenatal screening. Clin Chim Acta. 2021;521:206–211. doi:10.1016/j.cca.2021.07.015.
Subscribe to Clinical Diagnostics Insider to view
Start a Free Trial for immediate access to this article