Challenges and Opportunities in Lab Automation
As technology advances and testing demand grows, automation and AI are increasingly making their way into the clinical lab.
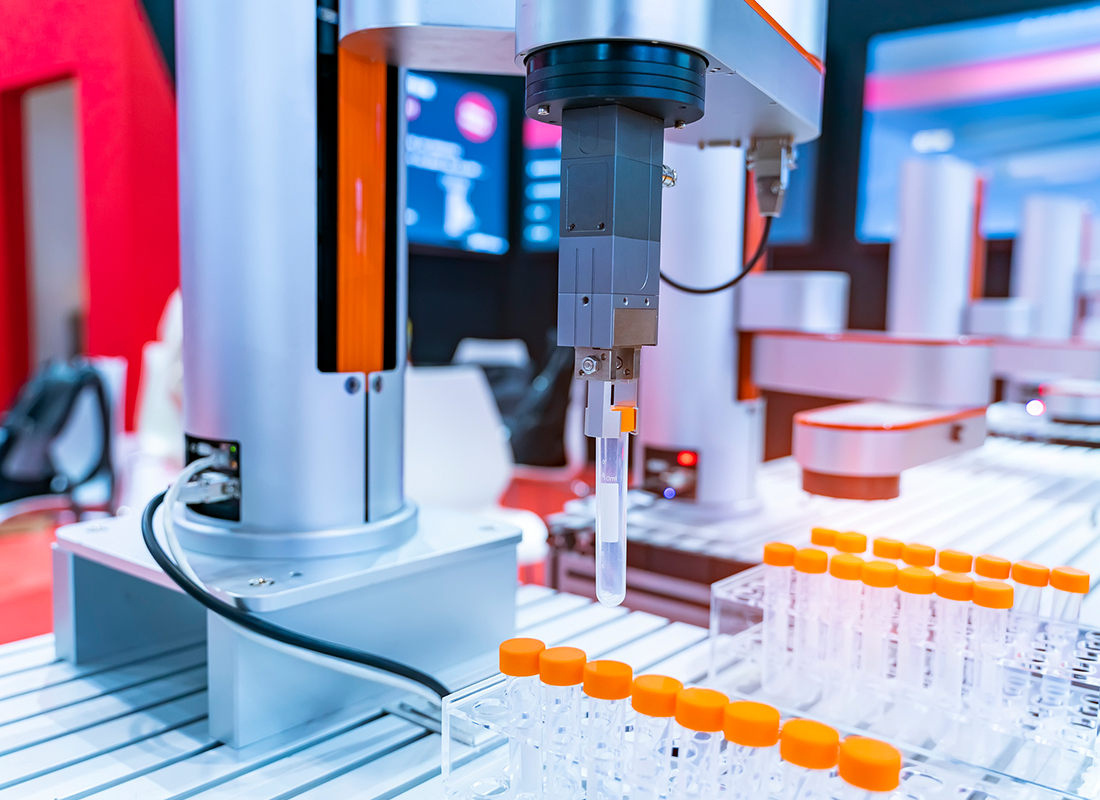
Although laboratory automation has been in development for over a century, the hype surrounding it has increased exponentially in recent years—thanks in part to rapid technological advancement and in part to staffing concerns in the clinical lab. But how powerful is lab automation really? What technologies are at the center of the hype today? And what are the potential benefits and drawbacks of making the move to embrace automation in the lab?
Where AI and automation meet
Artificial intelligence (AI) is a key focus in many labs’ automation planning. Currently, chemistry labs are leading the way in lab AI use, applying multimodal large language models to everything from protein design1,2 to drug discovery.3 In fact, some laboratories have even designed AI systems that can autonomously research, plan, and execute complicated experiments including chemical synthesis, liquid handling, coding, error correction, and optimization.4 But it’s not only in basic research that automation and AI meet; these technologies have a wide range of applications in the clinical laboratory as well.
Convolutional neural networks have been used to examine particles in urine samples5 and classify cells in peripheral blood smears6 for diagnostic purposes. In both cases, the AI’s automated analysis demonstrated high accuracy and precision (up to 96 percent on blood smears and 84 percent on urine particles) in timeframes as short as 72 ms per image. Recently, AI has been applied to more complex data, automating skin biopsy analysis,7 celiac disease diagnosis,8 drug testing,9 and even newborn screening.10 It’s clear that AI and automation go hand-in-hand—and that the role these technologies play in the clinical lab is only expanding.
Microbiology: pioneering total automation
Total laboratory automation is not yet possible for every discipline—but microbiology is one field in which most routine workflows can reap the benefits of automation. Automated streaking and inoculation, for example, can improve accuracy and yield over manual attempts11 and potentially reduce turnaround times and resource consumption by requiring fewer subcultures.12 Automated growth tracking not only increases timing precision,13 but can accelerate culture growth by minimizing the need to open incubators or remove plates for examination. Automated plate reading can further shorten turnaround times by reading multiple plates simultaneously and doing so as soon as they are ready, rather than waiting for a human to be available for the task. Increasingly, microbiology laboratories are exploring machine learning algorithms for diagnostic support, using them to automate tasks such as identifying bacterial vaginosis14 or detecting and staging malaria.15,16 The outcomes? Faster results for patients, increased productivity for labs, and the ability to function smoothly with fewer laboratorians, whose efforts can be directed toward non-automatable tasks.17,18
The cost equation
At first glance, automation seems like an obvious choice. Delivering rapid results while reducing consumption and freeing up staff is an appealing prospect for any laboratory—especially given that automation and AI can also lower the likelihood of diagnostic errors arising from the testing process.19 But introducing automation is not without its challenges and, as technology advances, so do the complications associated with its use.
Foremost among these is cost, particularly the capital costs associated with moving from manual to automated laboratory workflows. Although the prices of computing power and data storage have steadily decreased over time,20,21 implementing new systems remains costly and may require laboratories to make a strong business case based on corresponding reductions in staffing and supply costs, increased efficiency and productivity, and improved patient outcomes and satisfaction. When building the business case for automation, labs should also consider the operating costs of the equipment, the necessary training and infrastructure for its implementation, and the potential risks of bottlenecks or equipment failures.
A study of total laboratory automation implementations across labs of different sizes observed substantial cost savings in each setting (see Figure 1).18 The smallest lab, Willis-Knighton Laboratory (WKL), processed about 143,000 samples per year; both mid-sized labs, Hamilton Regional Medicine Laboratory Program (HRMLP) and Sutter Health Shared Laboratory (SHSL), processed about 600,000 samples per year; and the largest lab, TriCore Reference Laboratories (TC), processed about 775,000 samples per year.
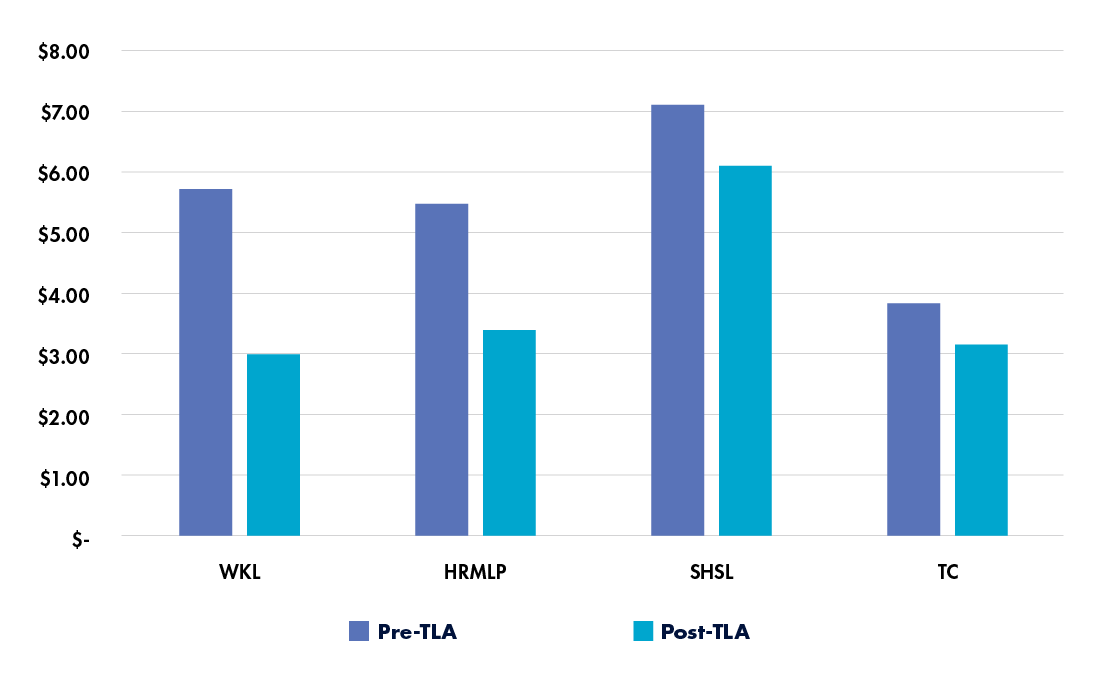
In all cases, the cost per specimen decreased following total laboratory automation. Some labs experienced higher daily labor costs, but these were offset by cost avoidance arising from large volume increases or by moving from off-site to on-site processing. Overall, the annual labor savings for the labs ranged from $268,000 to $1.2 million, demonstrating the potential for ongoing cost savings through automation.
Other challenges
Not every lab can automate. Some have specific sample preparation needs that still require manual handling; others lack the physical space or building features—for instance, ventilation or vibration reduction—required to accommodate the equipment and its needs. For these labs, partial or flexible automation solutions remain an option. Individual instruments can incorporate automated elements into otherwise manual workflows, whereas AI-based software can take on time-consuming tasks like cell counting and classification with minimal equipment requirements.
Staff training is needed for any move to automation; laboratorians should understand how automated systems work, how to operate them, potential error or failure points, and what to do if they experience problems or downtime. But will incorporating more automation into the lab workflow create a reliance on that technology? It’s possible that, as training focuses more on managing automated workflows and exposure to specific manual procedures decreases, those practical skills may be lost, which could create issues in the case of equipment downtime or when changing jobs. As a result, it’s important for both administrators and individual lab professionals to decide what learning and practice to prioritize and how best to protect the lab against a potential loss of necessary skills.
Finally, selecting specific instruments or software may limit the laboratory’s options for further automation or modification. This can impact not only future purchases, but also the lab’s physical layout and workflow design—so although committing to a single vendor or group may carry business or operational advantages, these must be balanced against the risks that can arise from a loss of interoperability.
There’s no single “right answer” for labs when incorporating automation, but it’s clear that the technology is becoming an ever-greater presence in the clinical laboratory. Reports of state-of-the-art automated and “self-driving” labs abound in clinical and translational spaces22,23 and publications showcase innovative software and devices for automating specific tasks.24,25 And, with frequent announcements of launches and regulatory approvals (see Table 1), laboratory automation is rapidly broadening its reach, accessibility, and customizability—so it’s up to labs to get on board.
Company | Date | Details | Link |
Abbott Laboratories | December 14, 2023 | FDA approval of GLP systems Track™ lab automation solution | http://tinyurl.com/g2abbottlabs |
Bio-Rad Laboratories | August 9, 2023 | 510(k) premarket authorization of IH-500 automated blood grouping and antibody test system | http://tinyurl.com/g2biorad |
Terumo BCT | July 27, 2023 | 510(k) premarket authorization of Reveos® automated whole blood processing system | http://tinyurl.com/g2terumo |
Diagnostic Grifols, S.A. | June 22, 2023 | 510(k) premarket authorization of Erytra Eflexis automated blood grouping and antibody test system | http://tinyurl.com/g2grifols |
Haemonetics Corporation | June 20, 2023 | 510(k) premarket authorization of NexSys PCS® Plasma Collection System with Express®Plus Technology automated blood separator | http://tinyurl.com/g2haemonetics |
Fresenius Kabi AG | April 21, 2023 | 510(k) premarket authorization of AMICUS Separator System automated blood separator | http://tinyurl.com/g2fresenius |
PerkinElmer; Oxford Immunotec | March 23, 2023 | FDA approval of Auto-Pure 24 and 20B platforms with T-Cell Select™ reagent kit for automated tuberculosis detection | http://tinyurl.com/g2peoi |
References:
-
- Madani A et al. Large language models generate functional protein sequences across diverse families. Nat Biotechnol. 2023;41(8):1099–1106. doi:10.1038/s41587-022-01618-2.
-
- Rapp JT et al. Self-driving laboratories to autonomously navigate the protein fitness landscape. Nat Chem Eng. 2024;1:97–107. doi:10.1038/s44286-023-00002-4.
-
- Mullowney MW et al. Artificial intelligence for natural product drug discovery. Nat Rev Drug Discov. 2023;22(11):895–916. doi:10.1038/s41573-023-00774-7.
-
- Boiko DA et al. Autonomous chemical research with large language models. Nature. 2023;624:570–578. doi:10.1038/s41586-023-06792-0.
-
- Liang Y et al. An end-to-end system for automatic urinary particle recognition with convolutional neural network. J Med Syst. 2018;42(9):165. doi:10.1007/s10916-018-1014-6.
-
- Acevedo A et al. Recognition of peripheral blood cell images using convolutional neural networks. Comput Methods Programs Biomed. 2019;180:105020. doi:10.1016/j.cmpb.2019.105020.
-
- Nofallah S et al. Automated analysis of whole slide digital skin biopsy images. Front Artif Intell. 2022;5:1005086. doi:10.3389/frai.2022.1005086.
-
- Wei JW et al. Automated detection of celiac disease on duodenal biopsy slides: a deep learning approach. J Pathol Inform. 2019;10:7. doi:10.4103/jpi.jpi_87_18.
-
- Wilkes EH et al. Using machine learning to aid the interpretation of urine steroid profiles. Clin Chem. 2018;64(11):1586–1595. doi:10.1373/clinchem.2018.292201.
-
- Peng G et al. Reducing false-positive results in newborn screening using machine learning. Int J Neonatal Screen. 2020;6(1):16. doi:10.3390/ijns6010016.
-
- Croxatto A et al. Comparison of inoculation with the InoqulA and WASP automated systems with manual inoculation. J Clin Microbiol. 2015;53(7):2298–2307. doi:10.1128/JCM.03076-14.
-
- Cheng CWR et al. Impact of BD Kiestra InoqulA streaking patterns on colony isolation and turnaround time of methicillin-resistant Staphylococcus aureus and carbapenem-resistant Enterobacterale surveillance samples. Clin Microbiol Infect. 2020;26(9):1201–1206. doi:10.1016/j.cmi.2020.01.006.
-
- Croxatto A et al. Towards automated detection, semi-quantification and identification of microbial growth in clinical bacteriology: a proof of concept. Biomed J. 2017;40(6):317–328. doi:10.1016/j.bj.2017.09.001.
-
- Wang Z et al. Deep neural networks offer morphologic classification and diagnosis of bacterial vaginosis. J Clin Microbiol. 2021;59(2):e02236-20. doi:10.1128/JCM.02236-20.
-
- Torres K et al. Automated microscopy for routine malaria diagnosis: a field comparison on Giemsa-stained blood films in Peru. Malar J. 2018;17(1):339. doi:10.1186/s12936-018-2493-0.
-
- Park HS et al. Automated detection of P. falciparum using machine learning algorithms with quantitative phase images of unstained cells. PLoS One. 2016;11(9):e0163045. doi:10.1371/journal.pone.0163045.
-
- Fontana C et al. Laboratory automation in microbiology: impact on turnaround time of microbiological samples in COVID time. Diagnostics (Basel). 2023;13(13):2243. doi:10.3390/diagnostics13132243.
-
- Culbreath K et al. Benefits derived from full laboratory automation in microbiology: a tale of four laboratories. J Clin Microbiol. 2021;59(3):e01969-20. doi: 10.1128/JCM.01969-20.
-
- Bakan E, Bakan N. Prevention of extra-analytical phase errors by non-analytical automation in clinical laboratory. Turkish J Biochem. 2021;46(3):235–243. doi:10.1515/tjb-2020-0483.
-
- Bergal A. 2019 recent trends in GPU price per FLOPS. AI Impacts. March 25, 2020. https://aiimpacts.org/2019-recent-trends-in-gpu-price-per-flops.
-
- McCallum JC. Historical cost of computer memory and storage. Our World in Data. March 1, 2023. https://ourworldindata.org/grapher/historical-cost-of-computer-memory-and-storage.
-
- London Health Sciences Centre. London’s hospitals are leading the way in clinical microbiology with a world-first installation of state-of-the-art automated laboratory technology. November 2, 2023. https://www.lhsc.on.ca/news/londons-hospitals-are-leading-the-way-in-clinical-microbiology-with-a-world-first-installation.
-
- Hazra A. U of T ‘self-driving lab’ to focus on next-gen human tissue models. U of T News. October 26, 2023. https://www.utoronto.ca/news/u-t-self-driving-lab-focus-next-gen-human-tissue-models.
-
- Arai Y et al. SAGAS: simulated annealing and greedy algorithm scheduler for laboratory automation. SLAS Technol. 2023;28(4):264–277. doi:10.1016/j.slast.2023.03.001.
-
- Namatame I et al. Screening Station, a novel laboratory automation system for physiologically relevant cell-based assays. SLAS Technol. 2023;28(5):351–360. doi:10.1016/j.slast.2023.04.002.
Subscribe to Clinical Diagnostics Insider to view
Start a Free Trial for immediate access to this article