Expert Q&A: Trends in Precision Medicine
Christian Olsen, a leader in R&D scientific software, discusses recent developments related to AI and solutions to current precision medicine challenges.
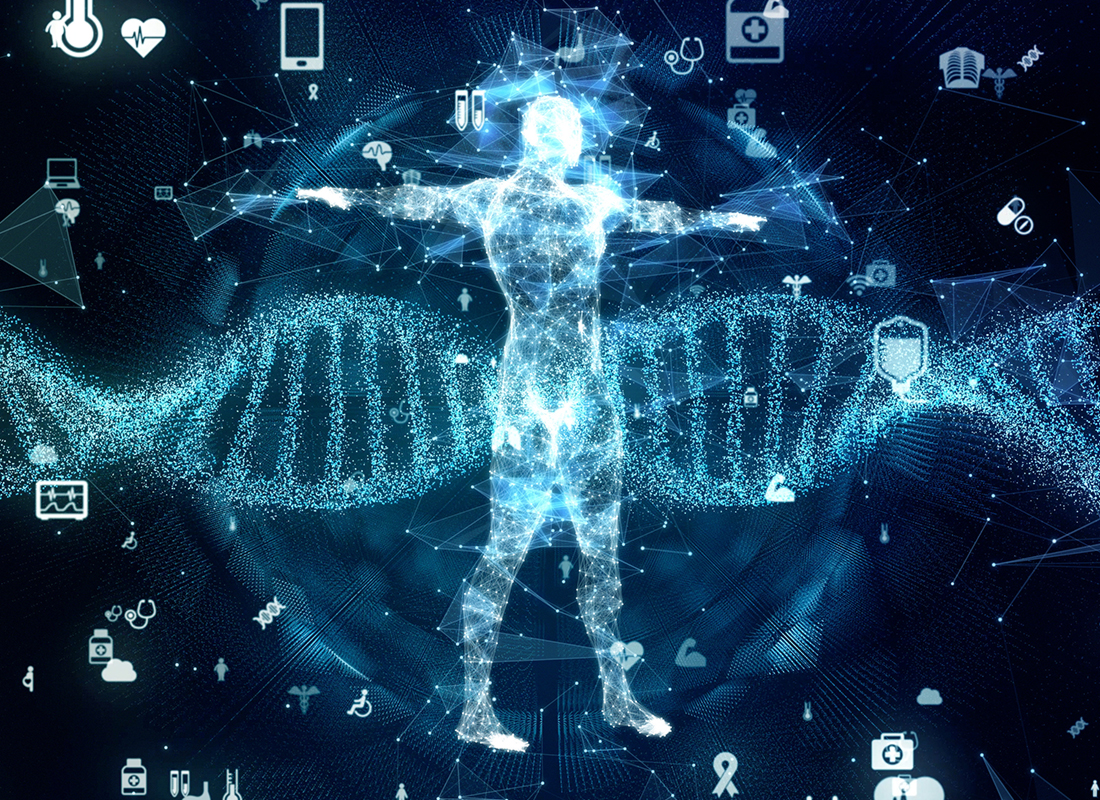
Subscribe to Clinical Diagnostics Insider to view
Start a Free Trial for immediate access to this article