Sepsis: Diagnosis on a Deadline
New molecular tests and diagnostic support technologies can help labs detect sepsis earlier, faster, and with greater accuracy
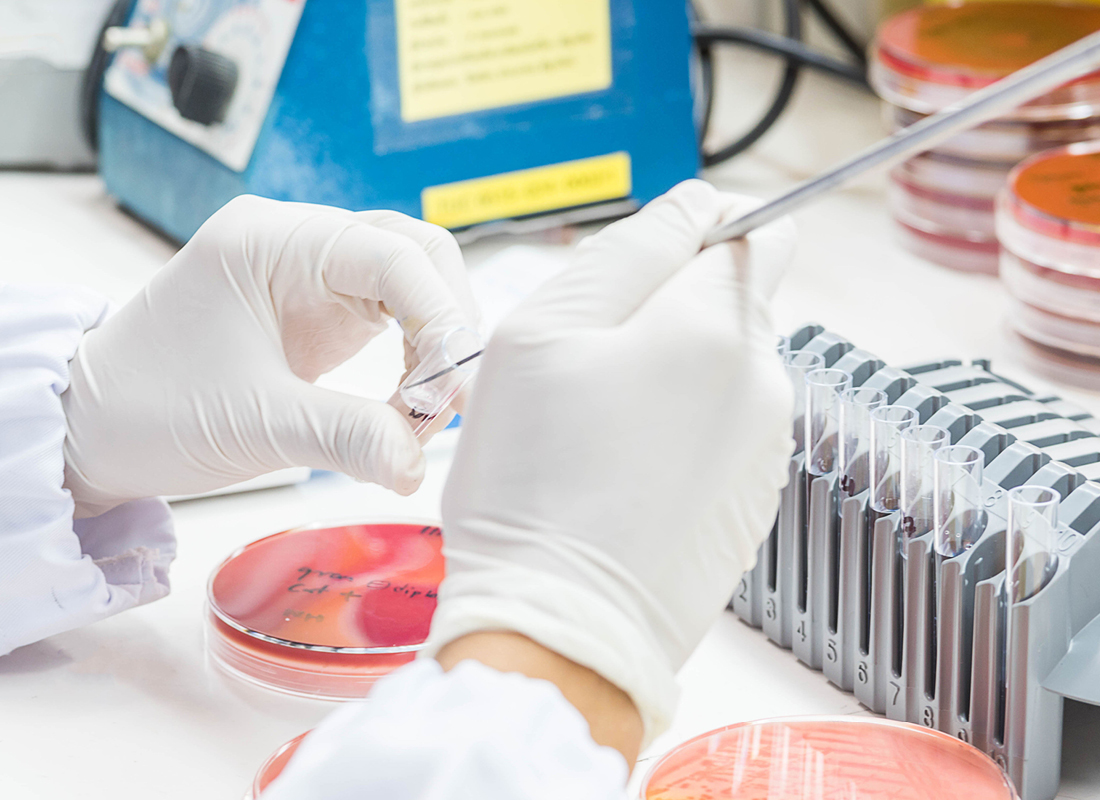
Rapid, accurate sepsis diagnosis is vital to saving lives—and, to achieve the best patient outcomes, every minute counts. Diagnostic delays of as little as one hour can increase the risk of progression to septic shock, reduce the likelihood of successful source control, and raise mortality rates.1 But the condition is often overlooked, especially if patients present with nonspecific symptoms—and conventional diagnostics such as blood cultures may take hours or days to yield results that can help guide treatment. As a result, the hunt for faster and more accurate sepsis testing is perpetual, with advanced molecular analyses and artificial intelligence (AI)-assisted diagnostics currently taking center stage.
Detecting pediatric sepsis
Young people are particularly vulnerable to infection, and sepsis is the leading global cause of childhood mortality.2 However, the international consensus criteria for pediatric sepsis diagnosis, established in 2005,3 were deemed inadequate due to low specificity, poor risk stratification capabilities, and a lack of concordance with clinical judgment.4 To remedy these issues, an international task force of critical care experts recently collaborated on a study of sepsis in health systems around the world.5 The result: new diagnostic criteria for pediatric sepsis and septic shock based on measures of respiratory, cardiovascular, hematological, and neurological dysfunction.6 The criteria also include redundancies and modifications to ensure usability and accuracy in low-resource settings. Applied retrospectively, they offered sensitive, specific diagnosis of sepsis and septic shock in over three million patients between 37 weeks and 18 years of age.
Recognizing the challenge nonspecific symptoms and markers present in pediatric sepsis, another research group reviewed existing literature in search of effective biomarkers. Using a multiomics approach, they identified a number of diagnostic and prognostic markers, as well as genes that increased sepsis susceptibility.7 Most require additional clinical investigation or validation but, taken together, offer promise for future biomarker panels that may be more sensitive and specific for predicting, diagnosing, and monitoring pediatric sepsis.
Rapid recognition yields results
Beyond pediatrics, a study from the University of Limerick considered the high risk of maternal sepsis, which—despite occurring in only 0.04 percent of US deliveries—causes 23 percent of all maternal deaths within six weeks of delivery.8 The study examined patients screened for sepsis using five different specimen types: blood, urine, or placental, vaginal, or throat swab.9 Placental swabs were the most effective in terms of pathogen yield, followed closely by high vaginal swabs; throat, blood, and urine samples each yielded potential pathogens in less than 10 percent of cases (see Figure 1).
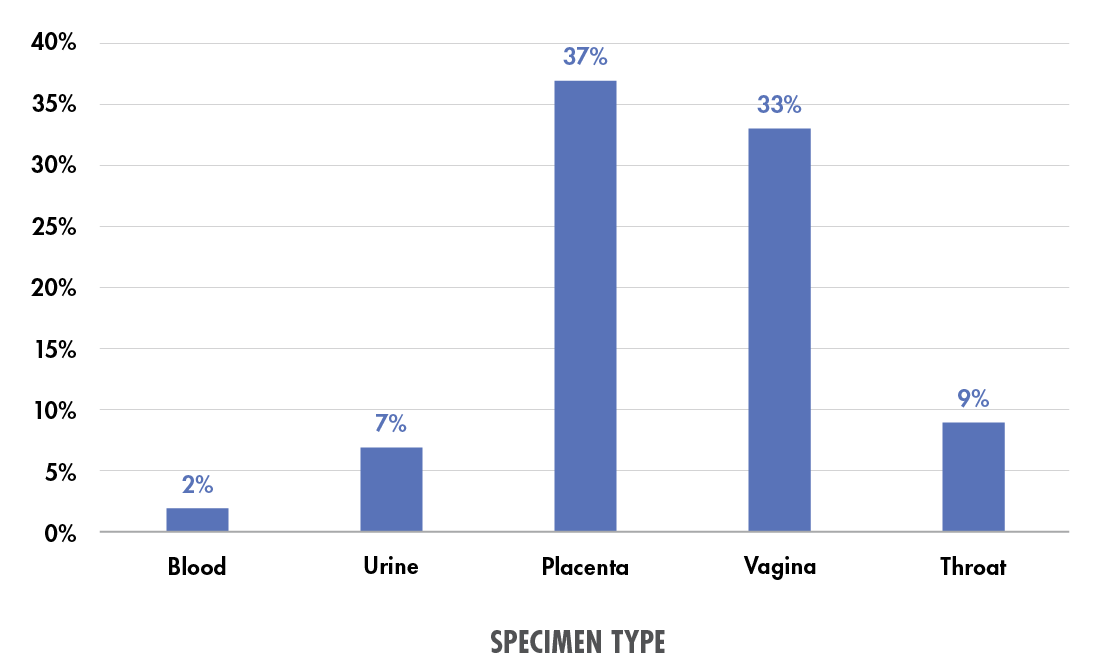
Figure 1. Percent of specimens of each type yielding potential pathogens in a maternal “septic screen.” Data from Powell et al. (2023).9
A new approach to molecular testing may further improve the speed and accuracy of sepsis diagnosis. Universal digital high-resolution melt (U-dHRM) analysis—an advanced technique for unbiased pathogen identification and quantification—allowed California-based researchers to deliver results in six hours (compared with 13.5–72 hours for positive and 120 hours for negative results by blood culture).10 The technique demonstrated 100 percent concordance with blood culture results in detecting infection and identifying the causative organism; however, the study’s small size necessitates more validation and further algorithm optimization to fully evaluate U-dHRM’s performance in the clinical lab.
Not all healthcare systems have the same access to testing, though—and it’s important to consider the most effective approach for each hospital. In Norway, recent research supported the use of simple molecular diagnostic tools for bloodstream infections in hospitals without dedicated microbiology departments.11 The tools enabled pathogen identification almost two days earlier than sending samples for conventional diagnostics and avoided the logistical challenges of long-distance transport. Furthermore, they prevented suboptimal or ineffective antibiotic treatment in over a quarter of cases, sparing patients unnecessary treatment and supporting better antimicrobial stewardship.
Algorithmic answers
For even earlier results, many healthcare systems now use machine learning algorithms that evaluate a range of clinical and laboratory indicators to predict the onset of sepsis or septic shock. Although promising in their ability to indicate emerging sepsis hours before symptoms arise,12 few studies have investigated the real-world benefits of these algorithms. That’s why a group of researchers looked at the before-and-after outcomes of implementing a deep-learning sepsis prediction algorithm in two San Diego emergency departments.13 The results? A 10 percent increase in sepsis bundle compliance, a 17 percent decrease in sepsis-related in-hospital mortality, and improvements in intermediate outcomes such as time to treatment, organ failure, and ICU transfer.
But many such algorithms never reach real-world implementation. A study of implementation processes revealed numerous barriers to the adoption of sepsis prediction algorithms, from clinician hesitancy to cost.14 However, the researchers also identified key enabling factors to help address these barriers during the implementation process (see Table 1).
Barriers | Solutions |
Clinicians: • Lack of clinician trust in algorithm • Concerns regarding overreliance • Confidence in own diagnostic skills; alerts occurring after suspicion of sepsis |
• Involve clinicians in selection and implementation • Clinical champions to advocate for algorithm • Position algorithm as “clinical decision support tool”; avoid mentions of “AI” • Report number of cases detected by algorithm, but missed by clinicians |
Training: • Lack of understanding or experience with machine learning systems • Lack of proficiency with software or hardware related to algorithm |
• Accessible information about algorithm, functions, and performance over time • Easy access to on-the-job training • Train all healthcare providers on “test version” of algorithm; use multiple training formats • Staggered deployment (if multiple sites) |
Alerts: • Alert fatigue • Alerts dismissed for incorrect reasons • Surveillance bias: more attention paid to high-risk or suspected sepsis patients • Delays in EHR data entry • Inherent limitations of EHR data |
• Alternative workflows during peak hours • Easy-read indicators such as color-coded alerts • Categorize patients in tabs or sheets based on status (triage, treatment, screened out) • Frequent communications, training, and improvement initiatives to ensure rapid data entry and alert response |
Logistics: • Concerns around algorithm retraining (feedback loops) • Cost to implement and maintain algorithm and associated systems • Bias toward or against specific systems |
• Alternative algorithm training methods • Track and monitor results and outcomes • Multidisciplinary team to select, implement, and oversee new algorithm(s) • Dedicated professional to integrate, maintain, and improve algorithm(s) |
With antimicrobial resistance on the rise and sepsis an ever-present healthcare threat, opportunities for faster, more targeted treatment and improved stewardship are vital. A move toward new diagnostic technologies, whether molecular or computational, can help clinical labs guide rapid treatment decisions for patients, prevent inappropriate or ineffective antibiotic use, and reduce the financial and human costs of sepsis.
References:
- Rüddel H et al. Adverse effects of delayed antimicrobial treatment and surgical source control in adults with sepsis: results of a planned secondary analysis of a cluster-randomized controlled trial. Crit Care. 2022;26(1):51. doi:10.1186/s13054-022-03901-9.
- Randolph AG, McCulloh RJ. Pediatric sepsis: important considerations for diagnosing and managing severe infections in infants, children, and adolescents. Virulence. 2014;5(1):179–189. doi:10.4161/viru.27045.
- Goldstein B et al. International pediatric sepsis consensus conference: Definitions for sepsis and organ dysfunction in pediatrics. Pediatr Crit Care Med. 2005;6(1):2–8. doi:10.1097/01.PCC.0000149131.72248.E6.
- Weiss SL et al. Discordant identification of pediatric severe sepsis by research and clinical definitions in the SPROUT international point prevalence study. Crit Care. 2015;19(1):325. doi:10.1186/s13054-015-1055-x.
- Sanchez-Pinto LN et al. Development and validation of the Phoenix criteria for pediatric sepsis and septic shock. JAMA. 2024; online ahead of print. doi:10.1001/jama.2024.0196.
- Schlapbach LJ et al. International consensus criteria for pediatric sepsis and septic shock. JAMA. 2024; online ahead of print. doi:10.1001/jama.2024.0179.
- Wang X et al. Multilevel omics for the discovery of biomarkers in pediatric sepsis. Pediatr Investig. 2023;7(4):277–289. doi:10.1002/ped4.12405.
- Hensley MK et al. Incidence of maternal sepsis and sepsis-related maternal deaths in the United States. JAMA. 2019;322(9):890–892. doi:10.1001/jama.2019.9818.
- Powell J et al. The microbial pathology of maternal perinatal sepsis: A single-institution retrospective five-year review. PLoS One. 2023;18(12):e0295210. doi:10.1371/journal.pone.0295210.
- Aralar A et al. Universal digital high-resolution melt analysis for the diagnosis of bacteremia. J Mol Diagn. 2024; online ahead of print. doi:10.1016/j.jmoldx.2024.01.013.
- Harboe-Sjåvik H et al. FilmArray (BCID2) provides essential and timely results in bloodstream infections in small acute care hospitals without conventional microbiology services. APMIS. 2024; online ahead of print. doi:10.1111/apm.13374.
- Islam KR et al. Machine learning-based early prediction of sepsis using electronic health records: a systematic review. J Clin Med. 2023;12(17):5658. doi:10.3390/jcm12175658.
- Boussina A et al. Impact of a deep learning sepsis prediction model on quality of care and survival. NPJ Digit Med. 2024;7(1):14. doi:10.1038/s41746-023-00986-6.
- van der Vegt AH et al. Deployment of machine learning algorithms to predict sepsis: systematic review and application of the SALIENT clinical AI implementation framework. J Am Med Inform Assoc. 2023;30(7):1349–1361. doi:10.1093/jamia/ocad075.
Subscribe to Clinical Diagnostics Insider to view
Start a Free Trial for immediate access to this article